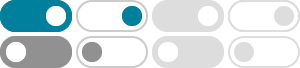
How come O (n) + O (logn) = O (logn) - Computer Science Stack …
Dec 2, 2018 · How come O (n) + O (logn) = O (logn)? When talking for example about an algorithm that has two operations. One of them takes O (n) and the other O (logn) and in the …
Is there a meaningful difference between O (1) and O (log n)?
Similarly even if an algorithm has O(log n) complexity, log n cannot possibly grow larger than about a hundred, so could be ignored as no larger than a small constant. So, is it really …
Is log n! = Θ(n log n)? - Computer Science Stack Exchange
Oct 17, 2015 · I tried: $\log (n!) = \log1 + \dots + \log n \leq n \log n \Rightarrow \log (n!) = O (n \log n)$. But how can we prove $\log (n!) = \Omega (n \log n)$ without Sterli...
O (Log n) Search - Array - Computer Science Stack Exchange
Apr 18, 2022 · This sort of got me thinking, what are the conditions under which you can do a O (log n) search for an element in an array in which all numbers are distinct? Clearly, you can …
How to solve T (n)=2T (√n)+log n with the master theorem?
OK but we already have two answers saying "change variables to cm c m, solve that recurrence and substitute to get T(n) = Θ(log n log log n) T (n) = Θ (log n log log n). So what does your …
Proof that n^2 = O (logn)? - Computer Science Stack Exchange
By definition, it means that there exists c,n0 c, n 0 such that for every n ≥ n0 n ≥ n 0, n2 <c log n n 2 <c log n, that is, n2 log n <c n 2 log n <c. Now you need to explain why this is absurd.
Can I simplify log(n+1) before showing that it is in O(log n)?
Had a question about the following: $$\\log (n+1) \\in O(\\log n)$$ Can the left side be simplified any further or do I need to just go ahead and find a c and n that hold?
notation - What is the difference between $\log^2 (n)$, $\log …
Jan 8, 2016 · Log^2 (n) means that it's proportional to the log of the log for a problem of size n. Log (n)^2 means that it's proportional to the square of the log.
What are the characteristics of a $\Theta (n \log n)$ time …
Your archetypical Θ(n log n) Θ (n log n) is a divide-and-conquer algorithm, which divides (and recombines) the work in linear time and recurses over the pieces. Merge sort works that way: …
n*log n and n/log n against polynomial running time
A large part of algorithmic complexity is to determine which algorithm is eventually faster, thus knowing that O(n^f) is faster than O(n/log n) for 0 < f < 1, is often enough.