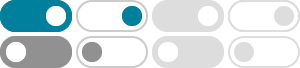
DFAN: : Dual Feature Aggregation Network for Lightweight Image …
Some lightweight SR methods solve this issue by directly designing shallower architectures, but it will adversely affect the representation capability of convolutional neural networks. To address this issue, we propose the dual feature aggregation strategy for image SR.
DFAN: Dual Feature Aggregation Network for Lightweight Image …
Jan 24, 2022 · To address this issue, we propose the dual feature aggregation strategy for image SR. It enhances feature utilization via feature reuse, which largely improves the representation ability while only introducing marginal computational cost. Thus, a smaller model could achieve better cost-effectiveness with the dual feature aggregation strategy.
To address this issue, we propose the dual feature aggregation strategy for image SR. It enhances feature utilization via feature reuse, which largely improves the representation ability while only introducing marginal computational cost. Thus, a smaller model could achieve better cost-e ectiveness with the dual feature aggregation strategy.
(PDF) DFAN: Dual Feature Aggregation Network for Lightweight Image …
Jan 24, 2022 · To address this issue, we propose the dual feature aggregation strategy for image SR. It enhances feature utilization via feature reuse, which largely improves the representation ability while...
dfan/single-image-surface-normal-estimation - GitHub
PyTorch implementation of the hourglass architecture proposed in "Single-Image Depth Perception in the Wild" by Chen, et al. in NIPS 2016. Adapted for surface normal estimation instead of relative depth estimation.
[PDF] DFAN: Dual Feature Aggregation Network for Lightweight Image …
Jan 24, 2022 · This work proposes the dual feature aggregation strategy for image SR, which enhances feature utilization via feature reuse, which largely improves the representation ability while only introducing marginal computational cost.
Dual-Feature Aggregation Network for No-Reference Image …
The proposed DFAN-IQA method exploits the pre-trained ViT and ResNet50 to extract attention features and semantic features from a distorted image, respectively. In addition, we have designed the AFA block and the SFA block.
Deep Feature Aggregation Network for Hyperspectral Anomaly …
The DFAN adopts an adaptive aggregation model (AAM), which combines the orthogonal spectral attention module (OSAM) with the background anomaly category statistics module. This allows effective utilization of spectral and spatial information …
I don't see anything when I close my eyes - dfan.org
Do you see things in dreams? Yep, I dream fine, in pictures and everything. Interestingly, I do start to see things as I am transitioning into a dream state. I can sometimes pull myself out as I'm falling asleep, and the vision fades instantly, but I can tell it was there. Can you draw? I'm quite good at drawing objects that are in front of me.
We propose an effective Dual-Feature Aggregation Network (DFAN) for NR-IQA by using the Convolutional Neural Networks (CNN) called ResNet50 and Vision Transformer (ViT).